Exploring the Landscape of Machine Learning: An In-Depth Analysis
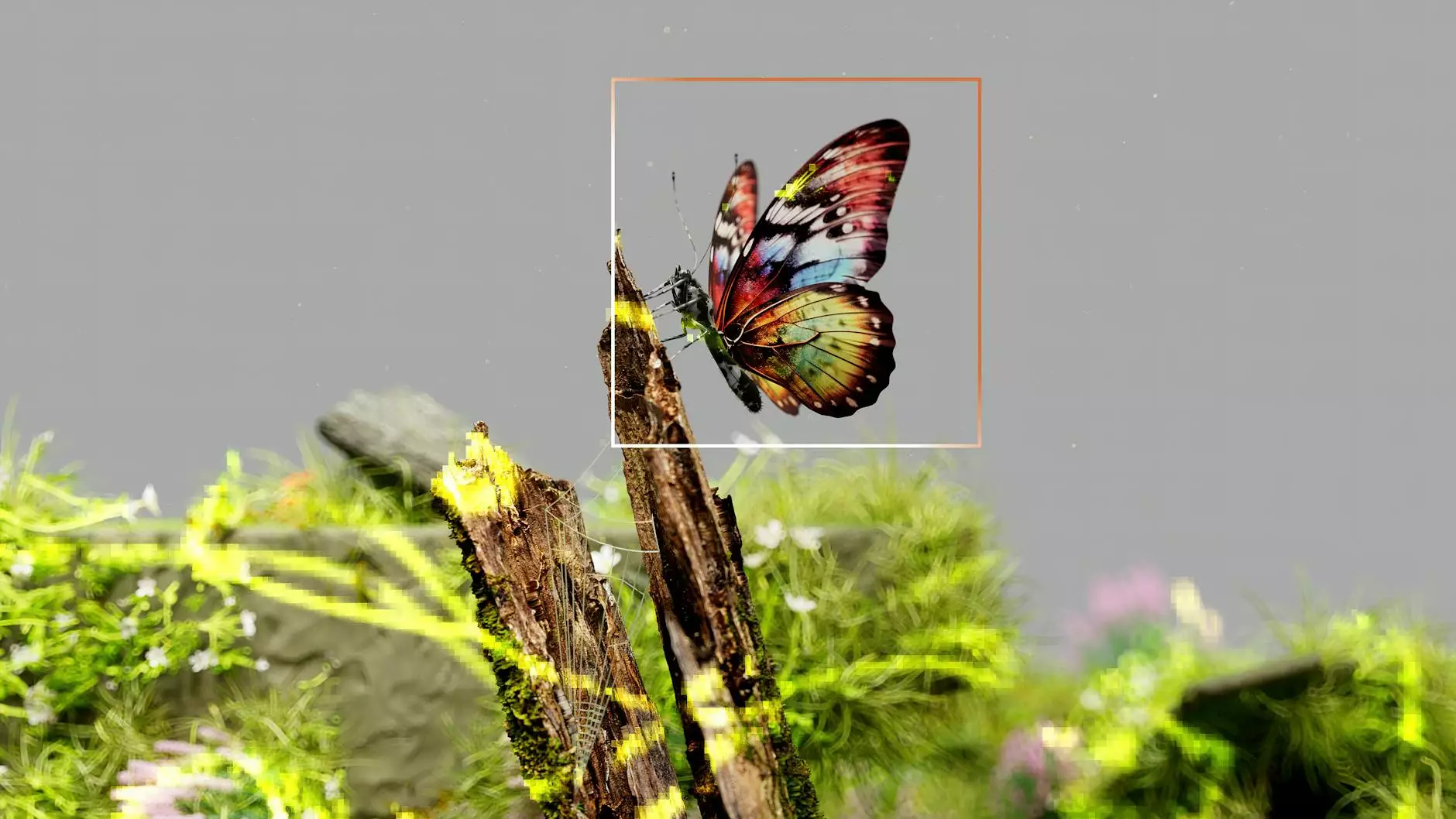
In today's digital era, machine learning stands at the forefront of technological advancement, revolutionizing industries across the globe. This article delves into the intricacies of machine learning, offering a thorough exploration of its principles, applications, and future potential. By understanding the core concepts and implications of this field, businesses can harness its power to drive innovation and gain a competitive edge.
What is Machine Learning?
At its core, machine learning is a subset of artificial intelligence (AI) that focuses on the development of algorithms that can learn from and make predictions or decisions based on data. Unlike traditional programming, where specific instructions are coded to achieve a result, machine learning allows systems to automatically improve their performance as they are exposed to more data. This flexibility and adaptability make it a powerful tool for businesses.
The Importance of Articles About Machine Learning
Staying informed through articles about machine learning is essential for professionals and organizations looking to thrive in a data-driven world. Here's why:
- Knowledge Building: Articles provide insights into the latest trends and techniques in machine learning.
- Industry Relevance: Understanding machine learning assists businesses in making informed decisions regarding technology implementation.
- Skill Development: Continuous learning through articles can help professionals improve their skills and stay competitive in the job market.
Key Concepts in Machine Learning
To understand the landscape of machine learning, one must grasp its fundamental concepts:
1. Types of Machine Learning
Machine learning can be broadly categorized into three types:
- Supervised Learning: This involves training a model on a labeled dataset, where the outcome is known. The model learns to predict future outcomes based on input data. Examples include regression and classification tasks.
- Unsupervised Learning: In this approach, the model learns from unlabeled data. It identifies patterns and relationships within the data without any specific target outcome. Clustering and association algorithms are prime examples.
- Reinforcement Learning: This type is about training models to make sequences of decisions by rewarding them for correct actions and punishing them for incorrect ones. It mimics a trial-and-error approach.
2. Algorithms in Machine Learning
Numerous algorithms are deployed in machine learning, each with unique strengths:
- Linear Regression: Useful for predicting a continuous dependent variable based on one or more independent variables.
- Decision Trees: A model that uses a tree-like graph to make decisions based on feature values.
- Support Vector Machines: These are used for classification tasks, which find the hyperplane that best separates different classes in the data.
- Neural Networks: These imitate the human brain's functioning and are particularly useful in complex tasks such as image and speech recognition.
Applications of Machine Learning in Business
Machine learning is reshaping various sectors by providing innovative solutions and enhancing decision-making processes. Here are a few key applications:
1. Customer Service Automation
Businesses utilize machine learning to power chatbots and virtual assistants, which can handle customer inquiries effectively and efficiently. This reduces operational costs and improves response times.
2. Predictive Analytics
Organizations leverage machine learning algorithms to analyze historical data and predict future trends, allowing for more informed decision-making. For instance, e-commerce platforms analyze purchasing patterns to recommend products to users.
3. Fraud Detection
In the financial sector, machine learning systems are employed to detect anomalies and patterns indicative of fraudulent activities, significantly enhancing security and protecting assets.
4. Personalized Marketing
Machine learning enables businesses to create highly personalized marketing strategies by analyzing customer behavior and preferences, thus increasing conversion rates and customer satisfaction.
Challenges in Implementing Machine Learning
Despite its advantages, integrating machine learning into business processes presents several challenges:
1. Data Quality and Quantity
Machine learning relies heavily on data, and poor quality or insufficient datasets can lead to inaccurate predictions and insights.
2. Skill Gap
There is a notable shortage of professionals with expertise in machine learning, making it difficult for organizations to find qualified personnel to implement and manage machine learning projects.
3. Ethical Considerations
Organizations must navigate ethical issues surrounding data privacy and algorithmic bias when deploying machine learning solutions.
The Future of Machine Learning
The potential of machine learning is boundless and is set to evolve rapidly. Here are some anticipated trends:
- Enhanced Automated Processes: We can expect more businesses to automate routine tasks using machine learning, leading to increased efficiency.
- Integration with Other Technologies: Machine learning will increasingly integrate with technologies like IoT, enhancing capabilities across various sectors.
- Improved User Experiences: As models become more sophisticated, businesses can offer more personalized and responsive services to consumers.
Conclusion: Embracing the Machine Learning Revolution
In conclusion, the landscape of machine learning is vast and dynamic, holding transformative potential for businesses. By engaging with articles about machine learning and staying abreast of the latest developments, organizations can unlock new opportunities and drive significant growth. Embracing this technology enhances not just operational efficiency but also customer experiences, paving the way for a more innovative future.
Ultimately, the world of machine learning is just beginning to unfold, and those who adapt to these changes will determine the business landscape of tomorrow. As you explore more about machine learning, remember that being informed is key to leveraging these advancements effectively.