Unlocking the Potential of Image Annotation for Machine Learning
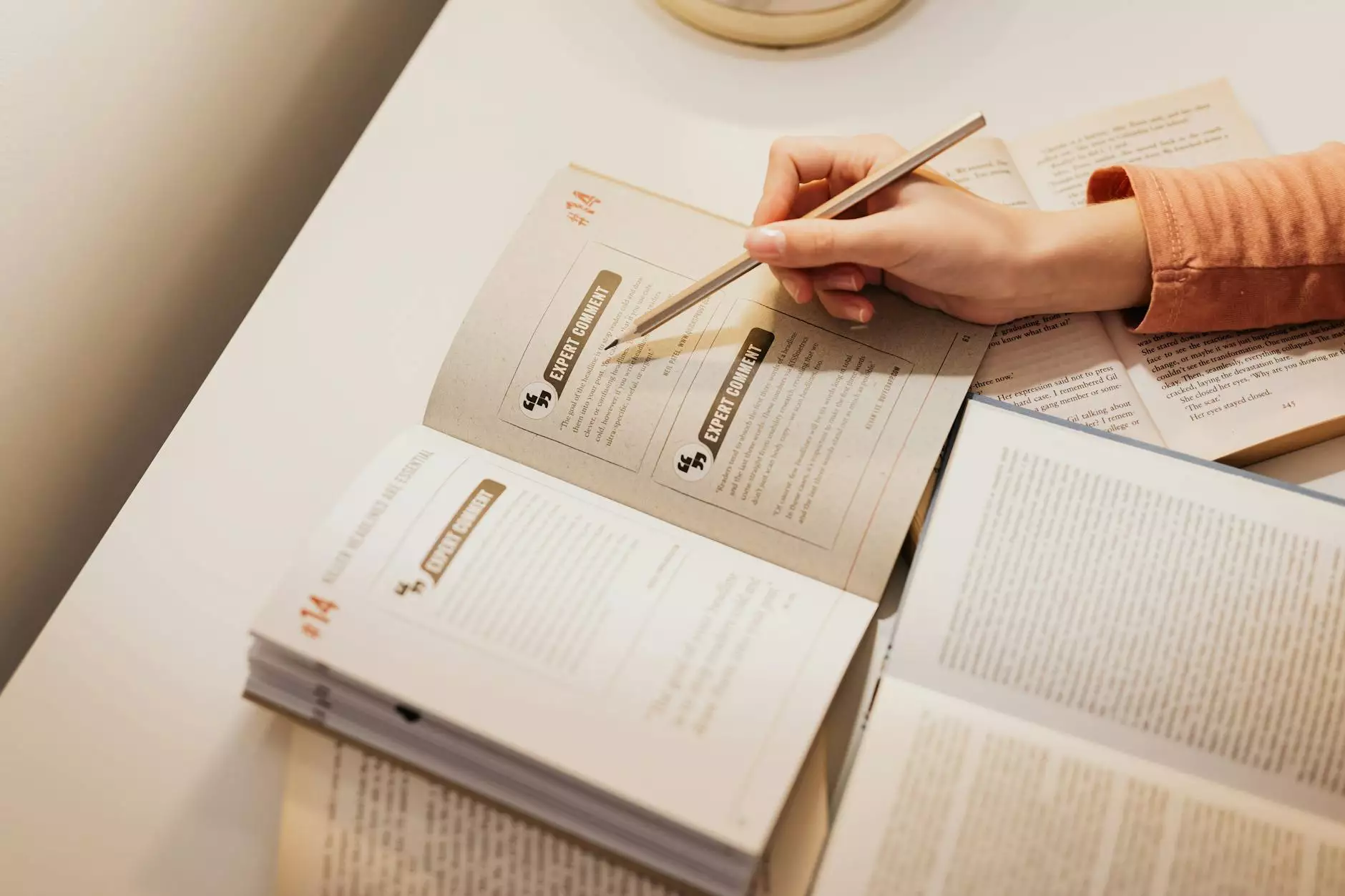
In the rapidly evolving domain of artificial intelligence (AI) and machine learning (ML), data quality stands as a paramount factor determining the success of any model. Among the various forms of data utilized in training machine learning algorithms, images hold a distinctive place. Consequently, the process of image annotation for machine learning emerges as a critical necessity, enabling machines to learn effectively from visual data.
Understanding Image Annotation
Image annotation refers to the process of labeling images with tags or bounding boxes to inform machine learning algorithms about the features present in those images. This makes it easier for algorithms to recognize objects, manage relationships between items, and deliver accurate predictions.
The Importance of Image Annotation
The significance of image annotation in the machine learning pipeline cannot be overstated. Here’s why:
- Enhances Model Accuracy: Well-annotated images allow machine learning models to learn representations with higher precision, leading to better predictive performance.
- Facilitates Supervised Learning: Many machine learning models depend on labeled data for training. Image annotation provides this labeled data, crucial for supervised learning.
- Supports Diverse Applications: Image annotation is vital in numerous applications, including object detection, image segmentation, facial recognition, and more.
The Process of Image Annotation
The process of annotating images involves several steps that ensure the creation of high-quality datasets for machine learning. Understanding these steps can dramatically improve your outcomes:
1. Choosing the Right Annotation Tool
Selecting an appropriate data annotation tool is the first step. Here, you can leverage platforms designed for effective annotation, such as Keylabs.ai, whose advanced features streamline the entire process.
2. Defining Annotation Types
Depending on the project requirements, choose the type of annotation needed:
- Bounding Box: Draw rectangles around objects to indicate their position and dimensions.
- Polygon Annotation: Useful for intricate shapes, allowing precise outlining of objects.
- Semantic Segmentation: Assign labels to each pixel in the image, ideal for image understanding.
- Keypoint Annotation: Identifying specific points in an image, critical for applications such as pose detection.
3. Training Annotators
Ensuring that data annotators are well-trained is essential for accuracy. Consistent annotation standards must be established to maintain data quality.
4. Quality Control Mechanisms
Implement quality control mechanisms to verify the accuracy of annotations. This can include peer reviews, where annotations are cross-checked by team members.
5. Iterative Feedback Loop
An iterative feedback loop can lead to continuous improvement in annotation quality. Using feedback from machine learning models can help refine the annotation process over time.
Best Practices for Image Annotation
If you want to maximize the efficacy of your image annotation for machine learning, adhere to these best practices:
- Use Clear Guidelines: Develop comprehensive annotation guidelines that detail how different scenarios should be handled.
- Employ Multiple Annotators: Utilize multiple annotators per image to reduce bias and enhance accuracy.
- Regular Training Sessions: Hold regular training workshops to keep annotators updated on best practices and tool usage.
- Leverage Automation: Consider using AI-based tools for automated annotations, which can speed up the process and improve consistency.
- Continuously Update Your Dataset: As your machine learning model evolves, regularly update the annotated images to include new data.
Keylabs.ai: Revolutionizing Your Data Annotation Process
At Keylabs.ai, our goal is to simplify and enhance the data annotation process with our robust data annotation platform. Our platform offers numerous features designed to improve the efficiency of your machine learning projects:
Comprehensive Toolset
Keylabs.ai provides a comprehensive set of tools for different types of annotations, allowing you to choose the best fit for your specific needs. From bounding boxes to semantic segmentation, our platform supports various techniques essential for effective data labeling.
Intuitive User Interface
Our user-friendly interface ensures that both seasoned professionals and newcomers can navigate the annotation process with ease. This usability can significantly reduce the learning curve, accelerating project timelines.
Scalability
Whether you are handling a small dataset or thousands of images, Keylabs.ai is designed to scale seamlessly with your project. This flexibility empowers businesses of all sizes to achieve their data annotation goals without distress.
Collaboration Features
Collaboration is simplified with our platform’s features that allow teams to work together efficiently. With real-time updates and annotations, all stakeholders remain on the same page.
Automated Quality Assurance
Our built-in quality assurance algorithms help maintain high standards of accuracy in image annotations. Leveraging machine learning techniques, we can identify anomalies and inconsistencies in annotations, ensuring a reliable dataset for training.
Applications of Image Annotation for Machine Learning
The applications of image annotation for machine learning are vast and varied, influencing numerous industries. Here are some of the most notable areas where annotated images play a crucial role:
1. Autonomous Vehicles
Self-driving cars rely heavily on image annotation to detect and categorize objects on the road. By identifying pedestrians, traffic signs, and other vehicles, these systems can make informed driving decisions.
2. Healthcare Diagnostics
In healthcare, annotated images (such as X-rays or MRIs) are used to train models for disease detection, aiding radiologists in identifying abnormalities with greater accuracy.
3. Retail and E-commerce
In the retail sector, image annotation facilitates visual search capabilities, enabling customers to find products using images rather than text queries, thus enhancing user experience and boosting sales.
4. Security and Surveillance
Security systems utilize annotated images to train models for facial recognition and suspicious activity detection, enhancing overall security measures in various environments.
5. Augmented and Virtual Reality
Annotated images pave the way for advancements in augmented and virtual reality by providing the necessary visuals for accurately overlaying digital content onto the physical world.
Future Trends in Image Annotation for Machine Learning
As technology continues to advance, so do the techniques and tools associated with image annotation for machine learning. Here are some emerging trends to observe:
1. Increased Automation
With the integration of machine learning into annotation tools, past methods of manual labeling are being increasingly complemented by automated systems that expedite the annotation process while ensuring accuracy.
2. Focus on Edge Cases
As models strive for higher accuracy, there is a shift towards annotating edge cases—scenarios where a model fails to perform well. By collecting and annotating these instances, organizations can enhance their model’s robustness.
3. Advanced Segmentation Techniques
Future image annotation strategies will likely utilize advanced segmentation methods that enable more precise understanding of image context, benefiting a myriad of applications.
4. Collaborative Annotation Platforms
The rise of cloud-based collaborative platforms will streamline the annotation process, allowing teams to work together in real-time, regardless of their geographical locations.
5. Ethical Considerations
As the ethical implications of AI and machine learning become a significant concern, there will be a stronger emphasis on transparency in the data annotation process, ensuring biases are minimized.
Conclusion
In conclusion, image annotation for machine learning is an essential component of the AI landscape, enabling machines to interpret and learn from visual data effectively. Understanding the nuances of the annotation process, adhering to best practices, and leveraging innovative platforms like Keylabs.ai can significantly enhance the quality of your datasets and drive the success of your machine learning initiatives. As technology continues to evolve, staying informed about trends and tools in this crucial domain will empower businesses to harness the full potential of their data.
With a commitment to excellence and innovation, companies like Keylabs.ai are perfectly positioned to lead the charge in the ever-evolving world of data annotation.