Unlocking Business Success with Advanced Data Annotation Tools and the Power of Bounding Box
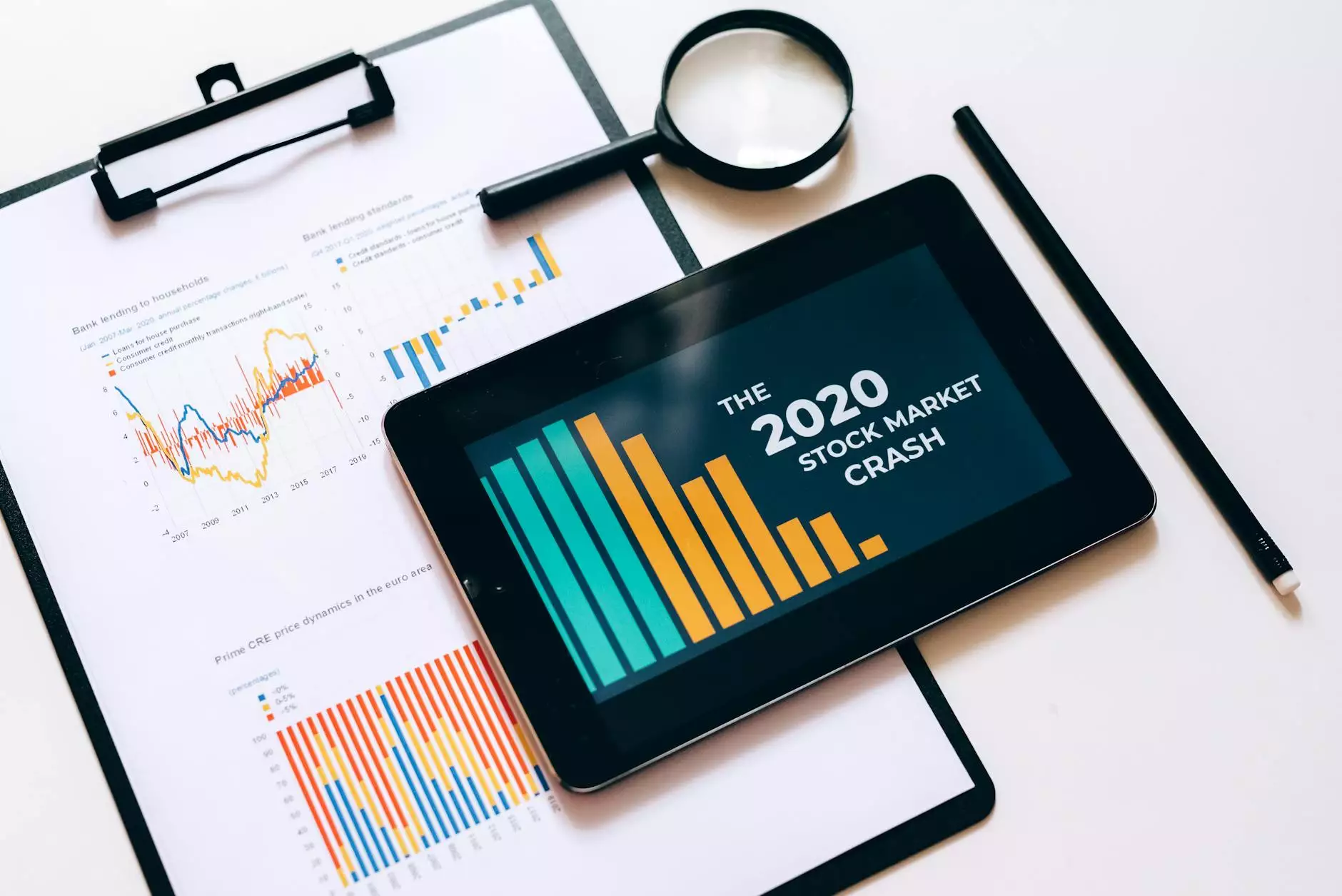
In today's rapidly evolving technological landscape, the integration of artificial intelligence (AI) and machine learning (ML) into business processes is no longer optional — it is a necessity for maintaining competitive edge and fostering innovation. Central to the success of AI models is the quality and precision of data annotation, which directly influences the accuracy and reliability of predictive algorithms. Among the myriad techniques employed in data labeling, the bounding box method stands out as a fundamental building block that enables machines to recognize and interpret visual data efficiently and effectively.
Understanding the Critical Role of Data Annotation in Business Innovation
Data annotation is the process of labeling raw data—be it images, videos, audio, or text—to make it understandable for machine learning algorithms. High-quality annotated data acts as the foundation upon which robust AI models are built, capable of performing complex tasks such as object detection, facial recognition, autonomous driving, and much more. Businesses that harness sophisticated data annotation platforms can unlock immense value, improving decision-making, operational efficiency, and customer engagement.
The Rise of Data Annotation Platforms: Revolutionizing Business Operations
As enterprises seek scalable solutions for their data labeling needs, data annotation platforms like keylabs.ai have emerged as leaders in the field. These platforms streamline the annotation process, offering intuitive interfaces, automation features, and collaborative tools that enable teams to produce high-quality labeled datasets rapidly. This acceleration plays a pivotal role in reducing time-to-market for AI-powered products and services.
The Bounding Box: The Cornerstone of Visual Data Annotation
The bounding box technique involves drawing a rectangular box around objects of interest within an image or video frame. This simple yet powerful method allows algorithms to identify the location and extent of objects, which is essential for tasks such as object detection, scene understanding, and autonomous navigation. The bounding box annotation provides structured, spatial information that enhances the training of machine learning models, especially convolutional neural networks (CNNs).
Benefits of Using Bounding Box Annotations in Business
- Improved Model Accuracy: Precise bounding box annotations enable models to learn better spatial representations, leading to higher detection accuracy.
- Efficiency in Data Labeling: The straightforward nature of bounding box annotation reduces time and costs involved in data preparation.
- Compatibility with Leading AI Frameworks: Most object detection algorithms, such as YOLO, Faster R-CNN, and SSD, rely heavily on bounding box labeled data.
- Scalability: Easily adaptable for annotating large datasets necessary for training industrial-scale AI solutions.
Implementing a Business-Driven Bounding Box Data Annotation Strategy
Effective utilization of bounding box annotations requires a strategic approach aligned with business objectives:
1. Identify Key Use Cases
Determine which business processes can benefit from computer vision. Examples include quality inspection, inventory management, driver assistance systems, and security surveillance.
2. Select the Right Annotation Platform
Opt for robust data annotation platforms like keylabs.ai that support bounding box labeling, collaboration, quality control, and automation features.
3. Train Skilled Annotators
Ensure data annotators are trained to accurately draw bounding box annotations, understanding object boundaries and contextual details to maximize data quality.
4. Leverage Automation and AI Assistance
Use semi-automated annotation tools that suggest bounding box labels, which annotators can verify and refine, significantly improving speed and accuracy.
5. Focus on Data Quality and Validation
Implement rigorous quality assurance protocols to minimize errors, as the performance of downstream AI models highly depend on annotation precision.
How keylabs.ai Empowers Business with Innovative Bounding Box Annotation Solutions
keylabs.ai specializes in providing next-generation data annotation tools and platforms that facilitate the creation of high-quality labeled datasets. Their solution encompasses:
- Intuitive Interfaces: Designed for both expert and novice annotators, enabling fast onboarding and efficient labeling.
- Automated & Assisted Labeling: Leveraging AI to suggest accurate bounding box annotations, reducing manual effort.
- Collaboration & Workflow Management: Tools that promote team collaboration, version control, and task assignment for large projects.
- Quality Assurance Modules: Built-in validation tools to ensure annotation accuracy and consistency across datasets.
- Scalable Infrastructure: Cloud-based solutions that support extensive annotation projects without compromising speed or performance.
Driving Business Growth through High-Quality Data and Bounding Box Annotation
By investing in advanced data annotation strategies, particularly the use of bounding box annotations, companies can significantly enhance their AI capabilities. This, in turn, leads to:
- Enhanced Customer Experiences: AI-driven visual recognition systems improve personalization and service delivery.
- Operational Efficiency: Automated inspection and monitoring reduce labor costs and minimize errors.
- New Revenue Streams: Developing innovative products powered by computer vision applications opens up new markets.
- Competitive Advantage: Superior datasets translate into more accurate models, positioning businesses ahead of competitors.
Future Trends in Business Data Annotation and Bounding Box Techniques
The landscape of data annotation and visual AI is continuously advancing. Key trends include:
- Automated & Semi-Automated Annotation: Continued development of AI-assisted tools to further reduce manual effort.
- 3D Object Detection: Extending bounding box concepts into three-dimensional space for applications like robotics and autonomous vehicles.
- Active Learning: Systems that intelligently select the most informative samples for labeling, optimizing resource use.
- Standardization & Best Practices: Unified guidelines to ensure high data quality and interoperability across platforms.
Conclusion: Transforming Business Potential with Precision Data Annotation
In conclusion, leveraging sophisticated bounding box annotation techniques within advanced data annotation platforms like keylabs.ai enables organizations to harness the full power of AI and machine learning. High-quality labeled datasets directly impact the effectiveness of AI applications, creating tangible business benefits such as improved customer satisfaction, operational excellence, and competitive differentiation.
As businesses continue to explore the transformative potential of computer vision and visual data analysis, investing in robust annotation processes will remain a critical success factor. The future belongs to those who recognize the significance of precise data labeling and leverage cutting-edge tools to achieve their strategic goals.
Harness the power of accurate data annotation today and position your business at the forefront of innovation with keylabs.ai.